First, the headlines: Geelong had the easiest fixture, GWS the hardest. But before we go any further, an important disclaimer: the Cats were so comfortably in far of everyone else, not even the league’s hardest fixture would have kept them from the minor premiership.
Home advantage is important, but not that important. If home advantage was as important as people say, all the left-column circles would be green and all the right ones would be red:
Games Won & Lost in 2022 (incl finals)
With Significant Home Advantage | Neutral-ish | With Significant Away Disadvantage | |||
---|---|---|---|---|---|
1 | ![]() | Geelong | WWWWLWWWWWW | WWWW | WWWLLWWWWL |
2 | ![]() | Sydney | LWWWWWLWWWW | WWW | WLWLLWLWWWL |
3 | ![]() | Brisbane | WWWLLWWWWWWW | W | LWWLWLWWWLLL |
4 | ![]() | Collingwood | WLWWWWLW | WWWWLLWWWWL | LLWWWL |
5 | ![]() | Fremantle | LWWLWLLWWWW | WW | WLLWWWDLWWW |
6 | ![]() | Melbourne | WLLLWL | WLWWWWLLWWW | WWWLWWW |
7 | ![]() | Richmond | WWWWDW | WWLWWLWLLWL | LLWLWL |
8 | ![]() | Bulldogs | WLWWLL | WWLWWWLW | WWLLWLLLL |
9 | ![]() | Carlton | WWWWLW | WLWLWWWLLL | WLWLLL |
10 | ![]() | St Kilda | WLLLW | LLWLWWWLLW | LWLWWLW |
11 | ![]() | Port Adelaide | LLWWWWLWWL | WLWL | LLLLLWLW |
12 | ![]() | Gold Coast | LLWWWLWW | LWW | LLLLLLWLLWW |
13 | ![]() | Hawthorn | WWWWLWW | LLLLLLLLLLLW | LWL |
14 | ![]() | Adelaide | LLLLWLWLWW | WL | LLLWWLLLLW |
15 | ![]() | Essendon | WWWLLL | LWWLLLLLLLW | LLLLW |
16 | ![]() | GWS | LLLLLLWWWW | LL | LLLLLLWWLL |
17 | ![]() | West Coast | LLLLWLLLLL | LL | LWLLLLLLLL |
18 | ![]() | North Melbourne | LLLLLW | LLLLLLLLW | LLLLLLL |
There is a bias there – home advantage is worth something – but it’s not a guaranteed ride to the Top Eight, or even a single extra win. You still actually have to be a good team.
(In the above table, “Significant Home Advantage” means games between interstate teams at a home ground, Geelong playing anyone at Kardinia Park, and Hawthorn or North dragging anyone off to Tassie.)
Of course, there are different degrees of home advantage. In Round 19 alone, we had:
- West Coast vs St Kilda @ Perth Stadium (WA) – an interstate game with fervent crowd support for the home team – that’s about as extreme a case as you’ll find, and good for 13.3 pts, by Squiggle’s model, which is generally calibrated to the level of crowd support
- Carlton vs GWS @ Docklands (Victoria) – an interstate game with good home crowd support, at a venue frequented fairly often by the away team – that’s 7.9 pts
- Brisbane vs Gold Coast @ the Gabba (Queensland) – two teams with smaller fan bases from the same state at one’s home ground – 2.8 pts
- Collingwood vs Essendon @ MCG (Victoria) – an extremely well-supported team hosts a very well-supported team at the Magpies’ home ground – 2.6 pts
- North Melbourne vs Hawthorn @ Bellerive Oval (Tasmania) – two teams in their secondary state, at a ground more often played by the Kangaroos – 2.0 pts
There’s a real hodge-podge of scenarios, which over the season shake out a bit like this:
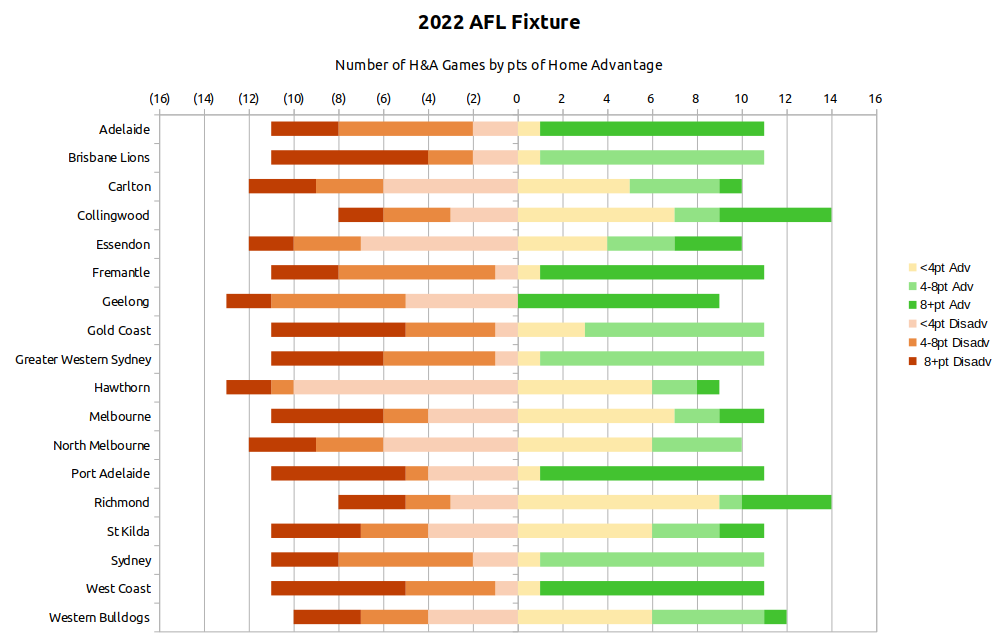
Don’t stare at that too long, though; there’s not much to be gleaned from it. The Squiggle model considers Collingwood and Richmond to enjoy many games of mild home advantage, by virtue of their large crowds at MCG games. The South Australian & West Australian teams usually have 10 games of extreme home advantage but fewer games of extreme disadvantage, as they revisit the same venues repeatedly (especially Docklands). NSW and Queensland teams essentially never create the same level of home advantage as the rest of the league, due to their lack of fan-filled cauldrons. And the Cats have a cauldron as well as warm fan support at many of their away games, which is a pretty handy setup.
Let’s now throw in Opposition Strength, because that’s the other big piece of the puzzle. As you know, each year the AFL carefully divides the previous year’s ladder into blocks of 6 teams, and assigns double-up games based on an equalisation strategy, so that weaker teams receive gentler match-ups.
Ha ha! We know that never works, since it only takes a couple of teams to shoot up or down the ladder to throw the whole thing out. But it may never have worked worse than this year, with Geelong, the eventual premier (and last year’s preliminary finalist) receiving quite gentle double-up games, while back-to-back wooden spooners North Melbourne faced a much sterner test. To some extent, this happens because teams can’t play themselves – you can’t fixture the wooden spooner against the wooden spooner – but still, things have not gone well when the premier has double-up games against the bottom 2 teams (representing 4 wins combined), while the bottom team faces both Grand Finalists, who have 34 wins.
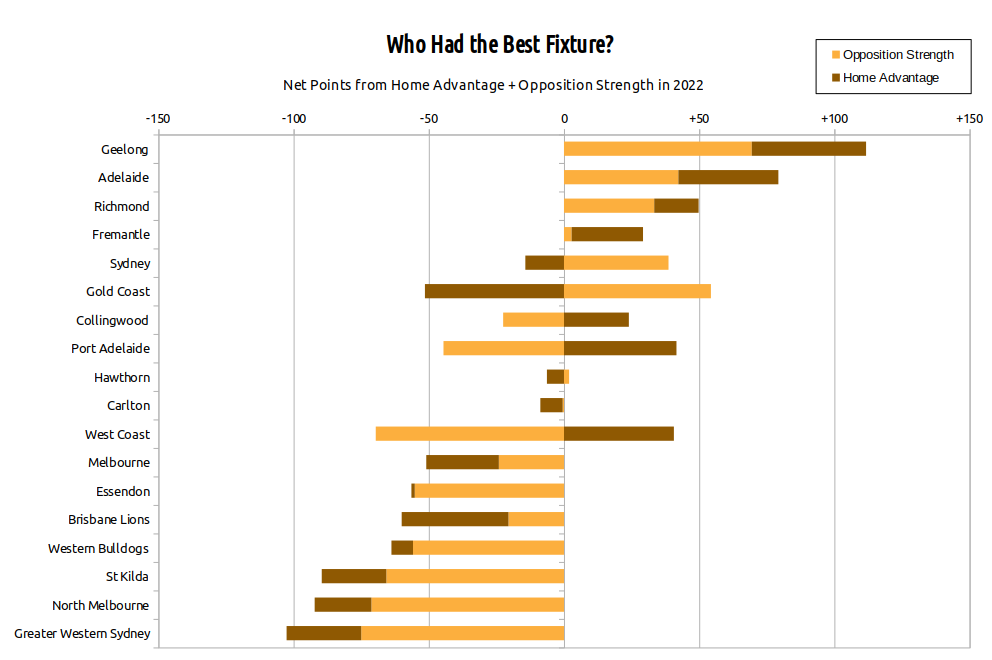
Overall, Adelaide did well out of the 2022 fixture – which, as a bottom-6 team, was at least to plan. Gold Coast, also lowly ranked in 2021, received a terrific set of double-up games, but lost it all to home advantage, as they hosted interstate teams at Carrara only 8 times while flying out 10 times themselves – and not just to familiar Docklands; the Suns were dispatched to every state plus the Northern Territory (twice), and even country Victoria.
St Kilda had terrible everything, as usual; St Kilda always have a terrible fixture, to the point where I’m starting to think it must be written into the AFL constitution. They hosted just 4 interstate teams (at Docklands, which their opponents visit often) while taking 6 interstate trips, including two to Perth, plus a bus to Kardinia. Their five double-up games – which should have been mild, as a middle-6 team – included both Grand Finalists, a Preliminary Finalist, and a Semi-Finalist. This combination of bad luck and bad design is very St Kilda, as was the Round 7 home game the Saints sold to play in Cairns and subsequently lost by a single point: a rare sighting of the case where a team’s unfair fixture really did cost them the match.
GWS also had four finalists in its five double-up games, and its fifth opponent was Carlton, who missed finals by a point. That’s enough for the Giants to take the booby prize for the worst set of match-ups.
Geelong’s bounty, while appreciated, I’m sure, was mostly wasted, since they finished two wins and percentage clear on top of the ladder, and were decidedly the best team in finals as well as the second half of the year in general (after Melbourne’s slide). It’s unlikely their fixture affected anything, and the Cats almost had a case for being dudded, escaping by 3 points against the Tigers in a home game played at the MCG, and by a goal against Collingwood in a home final at the same venue.
The 2023 AFL fixture will be released in the near future, and I have some thoughts. Chief among them: We are not actually achieving much equalization when we focus on the 6-6-6 system – which is obviously flawed and often produces the opposite effect – while ignoring systemic, completely predictable imbalances, such as:
- Poor teams sell home games.
- Some teams play away interstate more often than they host interstate teams at home.
- Some teams have many more games at the Grand Final ground – which doesn’t matter if you don’t make it, but can matter quite a lot if you do.
- Teams with smaller fan bases generate less home advantage.
- Geelong generate more home advantage playing any Melbourne-based team at Kardinia Park than they give away in the reverse match-up.
- Some teams have home games shifted to their opponents’ home ground.
To be fair, the fixture-makers do seem to be aware of most of the above, and I think they make some effort to avoid any of them becoming too egregious. But the priority is clearly the double-up games, which is the least predictable part of the equation. The result is that Docklands teams – especially St Kilda! – are almost guaranteed a bottom-4 fixture every year.
And maybe we can’t fix that; maybe the world isn’t ready for a fixture that provides kinder fixtures to poor teams with smaller fan bases. But it should be part of the conversation. Today, any talk of fixture fairness quickly shifts to how many times each team should play each other, and stops there, as if that’s the whole problem. It’s not: a 17-round fixture (or 34 rounds) won’t stop teams selling games, or being shifted to the MCG to face Richmond and Collingwood, or being sent to country Victoria; or, for that matter, being lucky enough to play a team when they have a bunch of outs versus when they don’t.
It’s a grab-bag of factors, and there’s no way to smooth them all out. Teams will inevitably have good fixtures and bad fixtures. But we can do better if we don’t rest the whole thing on 6-6-6 and the clearly wrong assumption that next year’s ladder will look just the same as today’s.